Stuck in the middle – mean vs. median
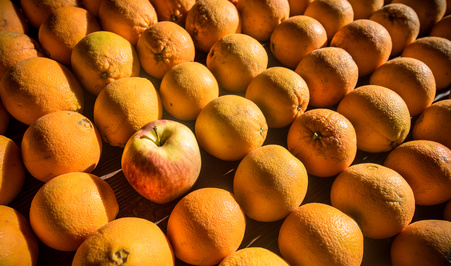
The mean value of numerical data is without a doubt the most commonly used statistical measure. Anyone who has a basic statistical background knows how to calculate the (arithmetic) mean – just sum up the individual values and divide the result by the number of values.
If, e.g., we talk about the average age of a group of people, we always refer to the mean of the individual age values. So there is also a general sense of understanding of this measure. The mean age can be interpreted as a single “representative” value describing the location of the age values of the people in this group. Therefore, in statistical language the mean is called a “location parameter”.
Sometimes the median is used as an alternative to the mean. Just like the mean value, the median also represents the location of a set of numerical data by means of a single number. Roughly speaking, the median is the value that splits the individual data into two halves: the (approximately) 50% largest and 50% lowest data in the collective.
Example
As an example, let us consider the following five measurements of systolic blood pressure (mmHg):
142, 124, 121, 151, 132.
The mean value is
(142 + 124 + 121 + 151 + 132) / 5 = 134
To calculate the median, we have to arrange the individual numbers according to their size, starting with the smallest:
121, 124, 132, 142, 151.
The median is defined as the value which is located in the middle, i.e. 132.
First we note that in this example mean and median do not differ very much, and that both can be seen as a reasonable representative value of the five individual measurements.
Secondly, we see why the word “approximately” was used for the description of the median in the above section: We cannot divide 5 numbers in two groups of exactly 50% of the data.
For an even number of values, however, we can: After sorting by size, the median is calculated as the mean of the two values that stand in the middle.
For
121, 124, 132, 142
the median is
(124 + 132) / 2 = 128
and exactly 50% of values are lower, respectively higher, than this number. In contrast to the situation of an uneven number of data values, the median is not necessarily a data value itself.
Mean vs. median: PROs and CONs
Now if both statistical measures, the mean and the median, are used to describe the location of a set of data, what about advantages and disadvantages?
As mentioned above, the mean is the more commonly used measure of the two. Moreover, it is the basis of many advanced statistical methods.
For example, the mean is needed to calculate the standard deviation, which is the most prominent measure to assess the variability in a set of data. And it is also needed for many statistical testing procedures, e.g. for the t-test.
But then, what are the advantages of the median?
To illustrate this, we return to the five systolic blood pressure values used before:
142, 124, 121, 151, 132.
We assume that 151 is a correct value, but that a device failure leads to the false measurement of 171. Let’s see what happens to mean and median?
The mean of the resulting five values now is 138 instead of 134, as calculated from the original data, thus showing a considerable effect of the incorrect measurement.
To derive the median, we sort the data again by size:
121, 124, 132, 142, 171.
As before, the value 132 is in the centre of the data row, so the median actually is unaltered by the false measurement.
That is why the median is called “robust against outliers“, whereas the mean actually is “sensitive to outliers“.
“Skewed” distributions
Another advantage of the median, associated with this kind of robustness, can be seen in “skewed” distributions.
An example for such a distribution in the context of an observational study is the time since the onset of a particular disease. In many cases, the date of diagnosis is close to the time of reporting, i.e. at or just a few days prior to the baseline visit. However, the study group often also includes patients who have been suffering from the disease for many years.
If we calculate the mean of the individual time spans since disease onset, such large values have an enormous impact, making the mean larger than the actual distribution of data would suggest.
The good news is that the outliers don’t have such an effect on the median. Therefore, here the median gives a more realistic picture of the data.
So which one should we use?
The best strategy is to calculate both measures.
If they are not too different, use the mean for discussion of the data, because almost everybody is familiar with it.
If both measures are considerably different, this indicates that the data are skewed (i.e. they are far from being normally distributed) and the median generally gives a more appropriate idea of the data distribution.
Picture: @ingaj /Fotolia.com