Unveiling the Veiled Influences: Bias in Observational Studies and Its Profound Impacts on Medical Research
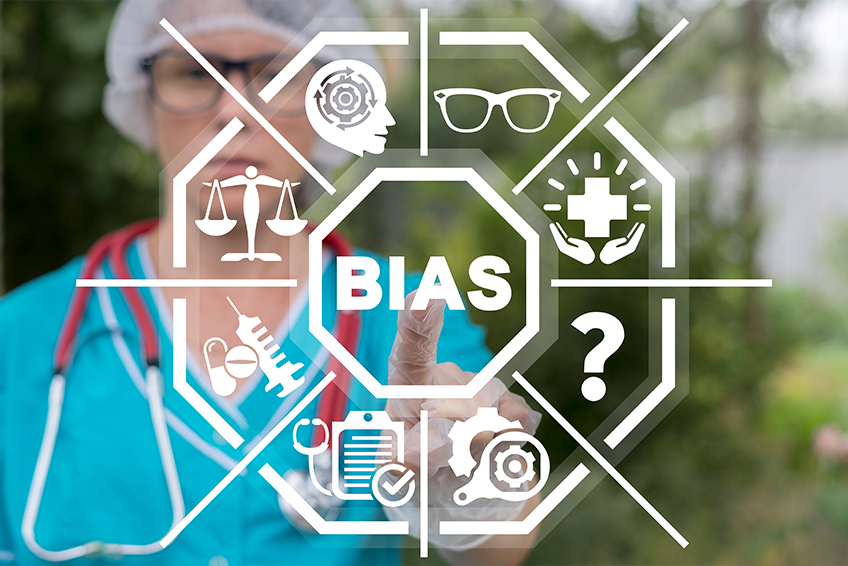
By Dr. Michael Obermeier
Observational studies serve as vital tools in uncovering insights into real-world healthcare scenarios. Yet, navigating the intricate landscape of scientific inquiry comes with its own set of challenges, and one of the most elusive adversaries is bias. In the catalogue of bias, developed by scientists of the University of Oxford, around 60 kinds of bias are described that might possibly influence clinical evidence (1). In this exploration, we focus on the most important ones and delve into the occurrence and profound impacts of the following four key bias types: Confounding, Selection Bias, Information Bias, and Reporting Bias (2, 3).
Confounding: Unseen Forces at Play
Imagine investigating the impact of several treatment choices on a specific disease. However, patients taking Medication A may already have a more severe form of the disease compared to patients with other treatment choices. This is where confounding enters the stage. It occurs when other factors influence both the choice of intervention and the study outcome, complicating the accurate assessment of causal relationships. Controlling these confounding variables is challenging but essential for drawing reliable conclusions.
There are mainly two ways this topic is addressed in the context of observational studies: design based approaches like restriction of population via eligibility criteria or statistical approaches that control confounders (3). The theory of causal inference and methods based on inverse probability weighting come here into affect (4–6). Both approaches (or a combination of those) are feasible and generally effective in controlling confounding effects. However, this type of bias will rarely fully be controlled as this would require complete identification and (correct) measurement of any possible confounders beforehand. In this context, the consultation of subject-matter experts as part of the planning process of a study is absolutely necessary and will surely help to reduce the impact of confounding (3). However, at the end there might still remain not identified or not fully measured confounders. Therefore, this sort of bias generally needs to be taken into account as a possible limitation in any research results.
Selection Bias: The Stealthy Distorter
Selection bias stealthily skews associations between intervention and outcome by including or excluding certain participants, timeframes, or events. For instance, studies focusing only on patients with milder symptoms may present an overly optimistic view of treatment effectiveness, distorting the true picture of its impact on diverse patient groups. A special kind of selection bias is the so called attrition bias caused by the occurrence of early terminations that might be associated with both, intervention and outcome (2). In order to minimise the impact of this bias type it is of importance to consider study dropouts in the analysis adequately.
Information Bias: The Precision Challenge
Information bias arises when data are inaccurately recorded or classified. This can result from measurement errors or imprecise data collection methods. If measurement errors are systematically linked to the intervention status observed in a study, it is referred to as differential misclassification. A special kind of differential misclassification is recall bias occurring in retrospective data collection when participants do not remember (correctly) previous events, possibly influenced by current conditions. The imperfect (and possibly selective) memory of unhealthy food intake in the past in a survey investigating the association between eating habits and overweight is an example for this kind of bias.
Bias based on imprecise data collection is known as detection bias or its special case observer bias: in this case, the measurement of the outcome is affected by the observer or the applied method and possibly associated with the intervention. The estimation of effects in animal experiments, for example, were shown to be significantly higher in those experiments where the outcome was assessed in a non-blinded way, i.e. where the rater was aware of the actual condition (7).
As the accuracy of information is crucial for the reliability of study findings, it is essential to omit information bias as far as possible: whenever feasible, prospective data collections are preferable to retrospective ones and standardized assessments of outcome measures are essential in any (observational) study. In addition, blinded outcome assessment would still more decrease the risk of information bias, although often not feasible in non-interventional study designs.
Reporting Bias: The Editorial Filter
Consider a study assessing the efficacy of a new therapy. Positive results may tempt researchers to publish their findings, while studies with negative outcomes might remain tucked away. The same is true when considering the role of publishers in this context: it seems to be easier and more tempting to publish significant findings than results without any proof character. This phenomenon is known as reporting bias (not only occurring in medical research!) and can lead to a distorted overall view of the available evidence. It emphasizes the need to share results impartially, irrespective of their direction or statistical significance, to ensure a balanced perception of research. The impact of reporting bias on scientific discussions is hardly quantifiable. However, from a methodological point of view it is enormous as it might even lead to wrong treatment decisions in specific cases. Furthermore, it undermines the concept of statistical significance and therefore the credibility of scientific research in general.
Due to its nature, reporting bias is hard to detect for single researches, but takes affect and becomes visible in the context of meta analyses, i.e. when information of various examinations is to be combined. Application of a systematic literature research, provision of funnel plots and other statistical methods are effective ways to preclude or at least to quantify its effect in meta analyses (8).
The Impact: Beyond the Data
The impacts of these biases extend far beyond statistical metrics. Inadequate control of confounding variables may portray medical interventions as more effective than they truly are. Selection bias distorts our understanding of disease trajectories, while information bias can lead to erroneous conclusions. Reporting bias, by favoring the publication of certain results, skews the collective understanding of interventions’ true effectiveness.
Navigating the Bias Minefield: A Call for Transparency
To address these challenges, transparency is paramount. Researchers must explicitly acknowledge potential biases in study protocols and employ clear strategies to minimize bias. Peer-review processes and scientific communities play a pivotal role in countering reporting bias by promoting comprehensive and unbiased dissemination of study results.
In conclusion, the examination of bias in observational studies underscores the necessity of understanding research limitations and conscientiously addressing these challenges. Only through this mindful engagement can observational studies make a genuine contribution to knowledge advancement and healthcare improvement.
Literatur
- University of Oxford, Center for Evidence-Based Medicine. Catalog of Bias. Verfügbar unter: https://catalogofbias.org/.
- Institut für Qualität und Wirtschaftlichkeit im Gesundheitswesen. Glossary, Types of Bias.
- Higgins J, Thomas J, Chandler J, Cumpston M, Li T, Page MJ et al., Hrsg. Cochrane handbook for systematic reviews of interventions. Second edition. Hoboken, NJ: Wiley-Blackwell; 2019. (Wiley Cochrane Ser).
- Liu T, Hogan JW. Unifying instrumental variable and inverse probability weighting approaches for inference of causal treatment effect and unmeasured confounding in observational studies. Stat Methods Med Res 2021; 30(3):671–86. https://doi: 10.1177/0962280220971835.
- Suarez D, Haro JM, Novick D, Ochoa S. Marginal structural models might overcome confounding when analyzing multiple treatment effects in observational studies. J Clin Epidemiol 2008; 61(6):525–30. Verfügbar unter: https://pubmed.ncbi.nlm.nih.gov/18471655/.
- Robins JM, Hernán MA, Brumback B. Marginal structural models and causal inference in epidemiology. Epidemiology 2000; 11(5):550–60. https://doi:10.1097/00001648-200009000-00011.
- Bello S, Krogsbøll LT, Gruber J, Zhao ZJ, Fischer D, Hróbjartsson A. Lack of blinding of outcome assessors in animal model experiments implies risk of observer bias. J Clin Epidemiol 2014; 67(9):973–83. https://doi:10.1016/j.jclinepi.2014.04.008.
- Schneck A. Examining publication bias-a simulation-based evaluation of statistical tests on publication bias. PeerJ 2017; 5:e4115. https://doi:10.7717/peerj.4115.
Picture: @ wladimir1804/AdobeStock.com